EN PT/BR
The truths of deepfakes
This text was originally published in Zum magazine n. 18, April 2020, and then updated and expanded for this book.
Artificial intelligence is out of the closet. It has abandoned the world of science fiction and is no longer exclusive to IT-field academics. It invaded startups, migrated to cell phones, took over the pornography industry, and announced new dimensions of image politics in the twenty-first century. Through machine learning processes and computer vision systems, the technology is disseminated in special effects, such as the rejuvenation techniques applied in Al Pacino and Robert de Niro in Martin Scorsese’s The Irishman (2019); it has once again resurrected Nicolas Cage in YouTube videos, and is the most feared polemic of 2020 elections, with the possibility of presenting Donald Trump making speeches from an opponent and vice versa.
After all, a preview of what might come next appeared in a viral video in April 2018, in which Barack Obama attacked the Black Panthers and cursed Trump (Mack, 2018), and reached the normal citizen’s world with the launch of the Chinese app Zao in September 2019, which allowed anyone to become a Hollywood star in seconds. In two days, it became a record-breaking download in the Chinese Apple Store, with millions of users.
The success was instantaneous, but so were the protests about violations of privacy since, at the time of its release, the app stated that it reserved the right to use the images and biometric information shared there. The complaints led the app to change this rule, and it is relevant that the new Chinese Internet legislation, announced in November, prohibits the use of non-explicitly stated AI resources, having the proliferation of fake news as one of its motivations. However, the most important part of this case was to highlight how AI-based image-creating technologies, especially deepfakes, can potentially become accessible and popular.
The term deepfake is a neologism that appeared in November 2017 on Reddit, a thematic discussions social network, as the nickname of a user and the name of a forum dedicated to applying deep learning technologies (where the “deep” in “deepfake” comes from) to synthetically exchange faces (face-swapping, the process of forgery that leads to the “fake” in “deepfake”) of porn actresses for celebrities. Banned from Reddit as a group in early 2018, the practice of deepfake is an absolute fact.
A survey conducted by the Dutch company Deeptrace, which develops algorithms for deepfake identification, shows that the number of deepfake videos grew almost 100% last year, jumping from 7,946 in December 2018 to 14,678 in December 2019. 96% of these videos are pornographic and reach about 135 million views. Misogynists, 100% of them target women. Among the non-pornographic videos, the phenomenon is inverted and focuses on men, usually politicians and corporate figures (Ajder et al., 2019).
Before we start to argument that there is nothing new in this, that Stalinism has made extensive use of adulterated photos, that Nazism and Fascism have defrauded countless others and that after Photoshop no one is surprised by manipulations of images anymore, it is good to emphasize: Deepfake is not collage, nor editing or dubbing. Deepfake is an image produced algorithmically, without human mediation in its processing, which uses thousands of images stored in databases to learn the movements of a person’s face, including the lips and their voice modulations, to predict how they could speak something they did not say.
In the installation In Event of Moon Disaster (2019), Francesca Panetta, creative director of MIT’s Center for Advanced Virtuality, in partnership with Halsey Burgund of the Open Documentary Lab at the same university, created a video in which President Richard Nixon reports the Apollo 11 disaster directly from the White House Oval Office. His speech was written by Bill Saphire and would be read in the event of an accident with the lunar mission which, as we know, did not happen. The motivation of this project, according to its authors, is to warn about the risks that deepfakes generate not only for present events, but also in that of historical revisionism (Panetta & Burgund, 2019).
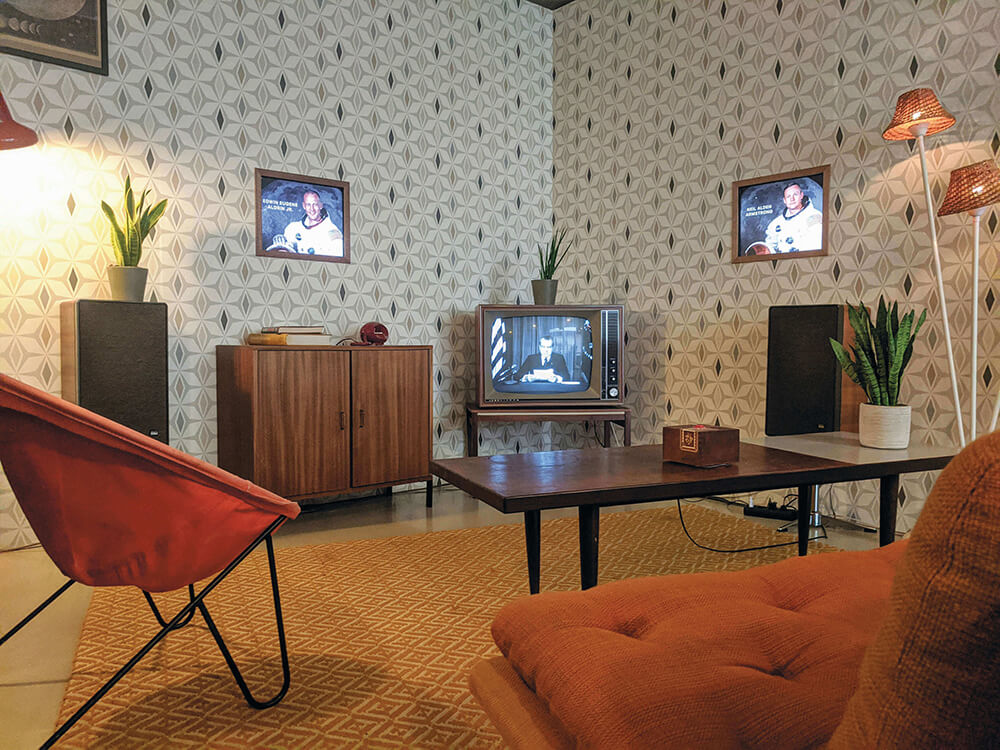
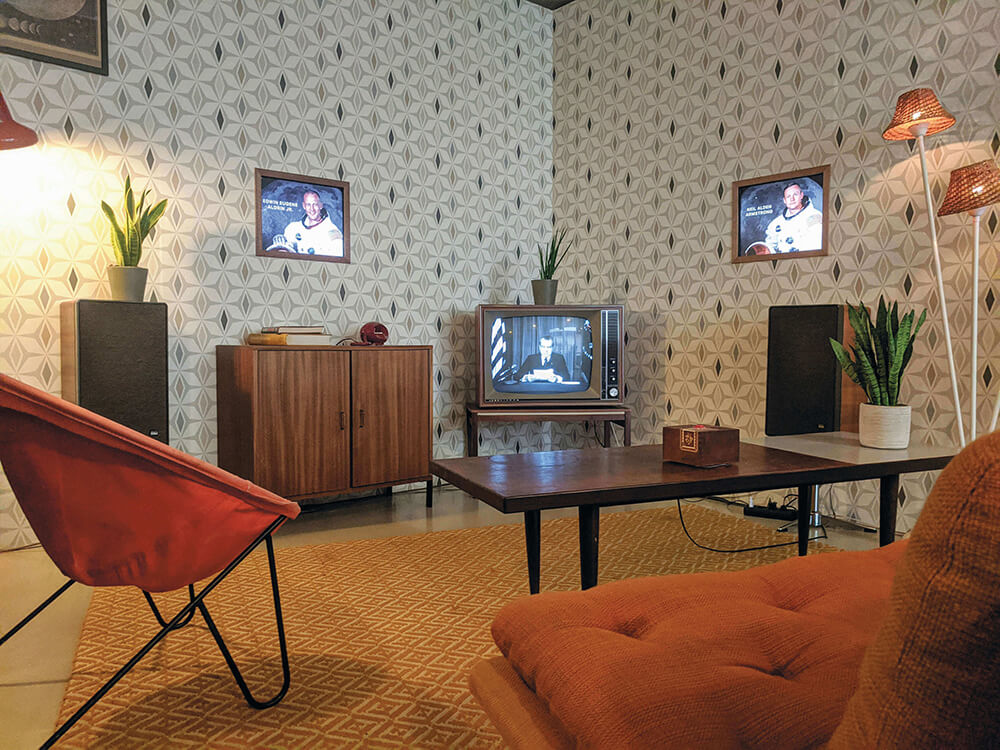
In Event of Moon Disaster, 2019. Installation featuring documentary with deepfake video, with the speech that President Nixon would make if a disaster had occurred during NASA’s Apollo 11 mission (1969).
The popularization of deepfake-creating resources and the ethical and political risks they entail led three of the largest technology companies—Microsoft, Amazon, and Facebook—to come together in a project, the Deepfake Detection Challenge, which ends in September 2020, to create deepfake identification and control features on their platforms. Hosted by Google’s platform Kaggle, the other big company in the team, it promises a $1 million prize to the winning team.
The union of these giants is enough to reveal the problem’s scale. Recent scandals, such as the role of Cambridge Analytica in Donald Trump’s election, and WhatsApp robots in the last presidential election in Brazil, are inescapable examples of the perverse relations between social networks, apps, and politics.
Although there are some indicators for recognizing a deepfake image (e.g. blurred backgrounds, unmatching earrings, strange microphone movements) the advances are fast and the tendency is for them to become more and more sophisticated. Moreover, after a video or a photo goes viral on the Internet, any subsequent action usually has merely palliative effects that are unlikely to compete with the damage already done.
Although they are fictional images, deepfakes are made from real images. They are built from large datasets and from neural networks, a computational architecture that tries to mimic the human brain (hence the ‘neural’ name). The algorithms search for patterns hidden in large amounts of information and use these patterns to group the data, classify them, and predict behaviors and actions.
This model marked a true revolution in the field of images with the development of GANs (Generative Adversarial Networks), a network architecture presented in 2014. In this architecture, two networks are pitted against each other, acting respectively as generators and discriminators. It is up to the first to create images and the second one to decide whether that image is real or false. From this cat and mouse game between algorithms, the discriminator learns how to recognize and classify the real images.
But the opposite also occurs. The more the discriminator learns to recognize fake images, the more the generator learns to trick it. This is the recipe behind a deepfake video and the reason celebrities and public personalities are more vulnerable to become the protagonist of a “deeply fake” video than other network users. The amount of images of these people available online is much larger than other ordinary users, providing more data for learning their gestures, facial expressions, and speech.
The ease of creating deepfakes increases as their methodologies and production capacity become more sophisticated. For example, the images from the website This Person Does Not Exist (2019), by Philip Wang, a senior software engineer at Microsoft, use a newer generation of artificial networks, the StyleGAN2. These networks extract, through algorithmic programming, information for style transfer (their aesthetic specificities, such as lighting, curves, contrasts, etc.) (Wang, 2019).
This Person Does Not Exist, 2019. Deepfake portraits algorithmically generated with StyleGAN2 neural networks
From the input of a facial image, the generator learns the distribution of the elements of a face and applies its characteristics to a new image. Unlike previous systems, which were unable to control what specific aspects of a face they would generate, this one can determine particular physical and facial attributes without changing any other. This results in a greater fidelity of identity and personal traits, such as hairstyles, eye shape and color, and face types.
Initially, the photos on this site are intriguing because they can make one believe that the people portrayed are real. They are also fascinating for being the first generation of realistic images that do not need the gaze, since they are synthesized by algorithms trained by machine learning systems. Thus, they write a new chapter in the history of post-photography, which had already discarded the need for the camera, a subject approached by several thinkers and photographers such as Juan Fontcuberta, whose Googlegrams series (2005) is a reference for the understanding of this emerging imaginary (Fontcuberta, 2007).
But there is something more disturbing about those pictures. Beyond the discussions about truthfulness, appropriation, and clashes between the human and the machinic—an eternal question of photography, as we have already learned from the theorists Raymond Bellour (1997) and Philippe Dubois (1993)— a new politics of images must be considered. We cannot ignore that all these new systems are produced by gigantic technology companies that monopolize countless sectors of contemporary social life. The GAN model was made by one of Google’s researchers, Ian Goodfellow. The StyleGAN was developed in the laboratories of Nvidia, the queen of GPUs (Graphics Processing Units, fundamental for the execution of games and videos), and a leader in the artificial intelligence market.
Digital images are not versions of chemical images made with new materials. They are computer images, carrying information that range from the geographic coordinates of where they were captured to the identity of who made them, their equipment, and how and when they were shared. More than a vision prosthesis, as Virilio called the camera, the devices of image capture today are, above all, devices of image extroversion. More and more linked to social networks such as Instagram, Snapchat, and TikTok, the cameras are meant for us to be seen and not to see.
The time of the camera as a framing and capture device is gone. After the digital, as indicated by Steyerl (2014), it became a projection device. Consequently, the image becomes the assumption of any intelligent surveillance system. This is indeed a trajectory that refers to the invention of photography, but as Jake Goldenfein pointed out in Public Books, no company that works with photography has risen to become a megacorporation. And we are not talking about its size and value. These corporations to which we refer are not only the owners of the main online services we use but the main players in the computer vision and data storage services market (Goldenfein, 2020).
And this dynamic essentially refers to the importance of patterns in today’s visual vocabulary. The whole neural network system depends on the construction of patterns. It is not by chance that all the portraits from This Person Does Not Exist have the same look and a poker face smile (or is “AI face” the new poker face?). Don’t deepfakes cry? Don’t they feel pain?
An experiment conducted by Bernardo Fontes, a researcher in the Group of Critical Experiments in Digital Infrastructures (GECID) of Inova-USP, shows the level of standardization embedded in computer vision processes. Bernardo downloaded 4,100 images generated by This Person Does Not Exist and separated three sets of 100, 500, and 1,000 images each, without repeating images. By overlaying the images, he expected a convergence in the points of the eyes, mouth, and nose. This is because the position of these organs is always fixed in the photos of This Person Does Not Exist. Its referential position is at the same x and y coordinates, regardless of whether the face appears frontally or in profile.
Composite overlays made by the GAIA / Inova-USP researcher Bernardo Fontes, using sets of 100, 500 and 1,000 distinct images of a set of 4,100 portraits from the This Person Does Not Exist website. This database reveals the standardization of the gaze in neural network
The amazing thing about the experiment was that, although the images were all different, the three sets resulted in almost identical images! It also draws attention, according to the study of another researcher from the same group, Lucas Nunes Sequeira, how fast the convergence appears, evidenced in the superposition of the first 100 images, when there is also the inclination to the dominance of the white skin pattern, revealing the matrices of social power embedded in the tsunami of pseudo-happy deepfake faces.
Made with datasets of real people’s images, they reproduce the racial and class dynamics that take place on the Internet. The image, as said, is machine-synthesized, coming from large sets that have been labeled by the precarious Amazon Mechanical Turk, an Amazon service that recruits remote workers for serial tasks at a minimal cost. In the case of StyleGAN2, the neural network behind This Face Does Not Exist, the turkers were responsible for building the Flickr-Faces-HQ database, used to build this network, and were in charge of removing images from statues, paintings or photos of photos, according to information in the repository of their code, stored in the GitHub platform.
Another point to consider in understanding the “AI’s smile” is that when they originate from datasets composed with images of the networks, they mirror how people present themselves online, often as heroes of their own lives where only success fits.
But deepfakes bring to light other complications of the normatization of the gaze that emerges with computational vision and that are not explained by recognized sociological or historical variables or the repertoire of art criticism. These complications refer to the productive chain that surrounds the cameras, less and less dependent on lenses and sensors and more on artificial intelligence, to image processing programs. Together they respond and model the standardized formatting of perspectives, colors, and points of view that are multiplied in various Instagram accounts, as ironized in Insta_repeat, a profile that conveys almost identical photos, in mosaics composed with pasteurized material that circulates in that social network (Scheffer, 2018-).
I agree that it is truly sensational when you take “that” picture that comes out all crooked and when you open it in the editor of your phone, it corrects itself and aligns everything. This is an indicator of the presence of computer vision in our daily lives and the ways we naturalize its rules in cultural expression. There will certainly be those who say that countless times the pattern does not correspond to what one wishes to register and it is possible to reverse it. I am sorry to inform you, however, that the tendency is that more and more “intelligent” cameras learn to capture the already corrected photos, making it difficult not to obey their prefabricated plans. We are living the paradoxical situation of potentially creating the richest and most plural visual culture in history, through the democratization of the media, yet we are diving into the limbo of a flattened gaze.
We only have to remember the selfie phenomenon to support this statement. After all, it changed forever the self-portrait angling, which ceased to be frontal, in correspondence with the camera on the tripod, and adapted to the viable angulation of capture with the cell phone in hand (between seven and seventeen degrees). Check out the Selfie City (2014), project of the pioneer of cultural data studies, Lev Manovich, if you don’t believe it (Manovich, 2014).
This process of naturalization of the machinic standard in visual culture, in what concerns deepfakes, has already begun. Snapchat and TikTok, the big current networks, use their resources to offer their users filters that allow them to put faces on new bodies. Still in a kind of funny way, with a truly false look. There are plenty of technologies to do more accurately what is done as entertainment on the networks and I bet it is just a matter of time for us to start using them recurrently.
Going further in the case of the selfies, the vicious and addictive circles of photos and videos that circulate on social networks, and especially the encoded standards on imaging devices (Steyerl, 2014), it is plausible to think that by overcoming the still persisting deepfake bugs, we will learn to live with them. Or rather: We will be trained by the machines to see them as “deeptrues.”
I definitely don’t think deepfakes are a momentary buzz. They must evolve and take other formats, but their “true core,” images produced with datasets controlled by standardized machine learning systems, is here to stay. But what about what is outside of the standard? What social place can it occupy? Is the deepfake the announcement of a new era of image eugenics?
REFERENCES
AJDER, Henry, Giorgio Patrini, Francesco Cavalli, e Laurence Cullen. 2019. “The State of Deepfakes: Landscape, Threats, and Impact.” Amsterdam.
BLLOUR, Raymond. 1997. Entre imagens: Foto, cinema, vídeo. Campinas: Papirus.
DUBOIS, Philippe. 1993. O ato fotográfico. Campinas: Papirus.
FONTCUBERTA, Joan. 2007. Datascapes: Orogenesis/Googlegrams. Paris: Photovision.
GOLDEFEIN, Jack. 2020. “Facial Recognition is Only the Beginning.” Public Books. 21 de Janeiro. Acesso em 17 de junho de 2020. https://www.publicbooks.org/facial-recognition-is-only-the-beginning/.
MACK, David. 2018. This PSA About Fake News From Barack Obama Is Not What It Appears. 17 de abril. Acesso em junho de 17 de 2020. https://www.buzzfeednews.com/article/davidmack/obama-fake-news-jordan-peele-psa-video-buzzfeed.
MANOVICH, Lev. 2014. Selfie City. Acesso em 17 de junho de 2020. http://selfiecity.net/.
PANETTA, Francesca, e Halsey Burgund. 2019. In Event of Moon Disaster. Acesso em 17 de junho de 2020. https://moondisaster.org/.
SCHEFFER, Emma. 2018-. “Insta_Repeat.” Instagram. Acesso em 17 de junho de 2020. https://www.instagram.com/insta_repeat/.
STEYERL, Hito. 2014. “Proxy Politics: Signal and Noise.” e-flux. Dezembro. Acesso em 17 de junho de 2020. https://www.e-flux.com/journal/60/61045/proxy-politics-signal-and-noise/.
WANG, Philip. 2019. This Person Does Not Exist. Acesso em junho de 17 de 2020. https://thispersondoesnotexist.com/.